朱玲英,徐露曼,黄雪旗,等.基于影像组学的脑胶质瘤Ⅲ、Ⅳ级分级预测模型构建[J].肿瘤学杂志,2024,30(10):833-839. |
基于影像组学的脑胶质瘤Ⅲ、Ⅳ级分级预测模型构建 |
Construction of a Prediction Model for Cerebral Gliomas Grading Based on Radiomics |
投稿时间:2024-07-19 |
DOI:10.11735/j.issn.1671-170X.2024.10.B006 |
|
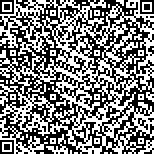 |
中文关键词: 脑胶质瘤 影像组学 Lasso回归 机器学习 |
英文关键词:cerebral glioma radiomics Lasso regression machine learning |
基金项目: |
|
摘要点击次数: 214 |
全文下载次数: 84 |
中文摘要: |
摘 要:[目的] 通过影像组学方法结合多种机器学习算法,开发一种高效、准确的脑胶质瘤Ⅲ级和Ⅳ级分级预测系统,以提高临床诊断和治疗的准确性及客观性。[方法] 收集台州市肿瘤医院和浙江大学医学院附属第二医院2013年3月至2018年6月间进行MRI检查的184例脑胶质瘤患者的影像数据。使用Python的pyradiomics库提取影像数据共107个影像组学特征。通过Lasso回归模型进行特征筛选,确定了最佳特征子集。应用朴素贝叶斯、支持向量机(support vector machine,SVM)、K近邻(K-nearest neighbor,KNN)和LightGBM四种机器学习算法进行胶质瘤分级预测。在此过程中,使用合成少数类过采样技术(synthetic minority oversampling technique,SMOTE)平衡训练数据,并对比不同模型的性能,选取最优模型。[结果] 未过采样的模型中,LightGBM在各项指标上表现最优,受试者工作特征曲线下面积为0.64,准确率为0.71,F1得分为0.80,灵敏度为0.82,特异度为0.44。经过SMOTE过采样处理后,各模型性能均有所提升,其中LightGBM的表现依然最优,受试者工作特征曲线下面积达到0.86,准确率为0.81,F1得分为0.81,灵敏度为为0.79,特异度为0.84。[结论] 通过影像组学特征提取和多种机器学习算法的应用,本研究成功开发了一种高效的脑胶质瘤分级预测系统。LightGBM模型在处理数据不平衡问题和胶质瘤分级预测任务中表现最佳,该系统可为临床诊断和治疗提供重要的辅助工具,具有潜在的临床应用价值。 |
英文摘要: |
Abstract:[Objective] To develop a prediction system for cerebral glioma grading based on radiomics. [Methods] The imaging data of 184 patients with cerebral gliomas who underwent MRI examination in Taizhou Cancer Hospital and The Second Affiliated Hospital, Zhejiang University School of Medicine from March 2013 to June 2018 were collected. A total of 107 radiomics features were extracted from image data using the pyradiomics library in Python. Lasso regression was employed to select the optimal subset of features. The machine learning algorithms Naive Bayes, Support Vector Machine (SVM), K-Nearest Neighbors (KNN), and LightGBM were applied for glioma grading prediction. During this process, synthetic minority over-sampling technique (SMOTE) was utilized to balance the training data, and the performance of different models was evaluated and compared. [Results] Among the models without over-sampling, LightGBM exhibited the best performance across various metrics, with an AUC(area under the ROC curve) of 0.64, accuracy of 0.71, F1 score of 0.80, sensitivity of 0.82, and specificity of 0.44. After applying SMOTE over-sampling, the performance of all models improved, LightGBM still achieved the best results, with an AUC of 0.86, accuracy of 0.81, F1 score of 0.81, sensitivity of 0.79, and specificity of 0.84. [Conclusion] Through the application of radiomics feature extraction and multiple machine learning algorithms, this study has successfully developed an efficient system for predicting glioma grades. The LightGBM model demonstrated superior performance in handling data imbalance and glioma grading tasks, showing substantial clinical application value. |
在线阅读
查看全文 查看/发表评论 下载PDF阅读器 |
|
|
|