李建东,谢宝文,贾文廷,等.基于3D残差U-Net的放射治疗剂量分布预测[J].肿瘤学杂志,2023,29(2):145-149. |
基于3D残差U-Net的放射治疗剂量分布预测 |
Radiotherapy Dose Distribution Prediction Based on 3D Residual U-Net Model |
投稿时间:2022-10-12 |
DOI:10.11735/j.issn.1671-170X.2023.02.B010 |
|
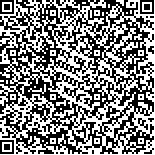 |
中文关键词: 放射疗法 剂量分布预测 深度学习 U?鄄Net 残差网络 |
英文关键词:radiotherapy dose distribution prediction deep learning U-Net residual network |
基金项目:国家自然科学基金(12005190);浙江省放射肿瘤学重点实验室开放项目(2022ZJCCRAD08) |
|
摘要点击次数: 427 |
全文下载次数: 127 |
中文摘要: |
摘 要:[目的] 针对放射治疗中剂量分布预测结果精度低的问题,提出一种基于深度学习技术的肿瘤放疗三维剂量分布预测模型。[方法] 选择130例宫颈癌患者数据,包括CT影像和靶区文件。通过基于卷积神经网络和残差块的深度学习U-Net模型,用于自动提取调强放疗放疗计划的CT影像、靶区和危及器官解剖结构群的多尺度和多层次特征图并进行三维剂量分布预测。采用定量分析方法,包括实际剂量与预测值之间的误差包括最大剂量(Dmax)、平均剂量(Dmean)、D99、D98、PTV56、PTV63和PTV70等。[结果] 对于宫颈癌,根据检测结果绘制剂量-体积直方图,直接反映了真实剂量与预测剂量的差异,实际误差满足基本要求。[结论] 通过深度学习模型的训练回归,拟合了调强放疗计划特征图参数与三维剂量分布之间的复杂非线性函数关系。在实际临床应用中,该训练模型可以准确预测新患者的个性化三维剂量分布。 |
英文摘要: |
Abstract: [Objective] To develop a three-dimensional dose distribution prediction model for tumor radiotherapy based on depth learning technology. [Methods] The data of 130 patients with cervical cancer were reviewed, including CT images and target files. Through the deep learning U-Net model based on convolutional neural network and residual block, the CT images of intensity modulated radiotherapy, the multi-scale and multi-level characteristic maps of target areas and anatomical structures of endangered organs were automatically extracted, to predict the three-dimensional dose distribution. Quantitative analysis method was adopted for the error between actual dose and predicted value, including the maximum dose (Dmax), average dose (Dmean), D99, D98, PTV56, PTV63 and PTV70. [Results] Through the training regression of the depth learning model, the complex nonlinear functional relationship was fited between the IMRT plan characteristic map parameters and the three-dimensional dose distribution. For cervical cancer, the DVH map was drawn according to the detection results, which directly reflected the difference between the real dose and the predicted dose, and the actual error met the basic requirements. [Conclusion] The training model developed in this study can accurately predict the personalized three-dimensional dose distribution of new patients. |
在线阅读
查看全文 查看/发表评论 下载PDF阅读器 |