陈 亮,巴哈提.CT纹理分析在区分非小细胞肺癌组织学亚型中的应用价值[J].肿瘤学杂志,2023,29(2):123-128. |
CT纹理分析在区分非小细胞肺癌组织学亚型中的应用价值 |
Application Value of CT Texture Analysis in Differentiating Different Histological Subtypes of Non-Small Cell Lung Cancer |
投稿时间:2022-08-15 |
DOI:10.11735/j.issn.1671-170X.2023.02.B006 |
|
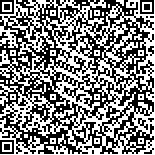 |
中文关键词: CT 纹理分析 非小细胞肺癌 组织学亚型 集成学习 |
英文关键词:CT texture analysis non-small cell lung cancer histological subtypes integrated learning |
基金项目:新疆维吾尔自治区卫生健康委员会科研基金项目(20210728) |
|
摘要点击次数: 480 |
全文下载次数: 181 |
中文摘要: |
摘 要:[目的] 探讨CT纹理分析在区分肺鳞状细胞癌(squamous cell carcinoma,SCC)和腺癌(adenocarcinoma,AD)中的应用价值。[方法] 2020年3月至2022年3月从新疆医科大学第一附属医院共收集70例术后确诊的NSCLC患者资料。将患者随机分配到训练队列(n=50)和测试队列(n=20)。从每例患者CT 数据的瘤内和瘤周区域中提取了7类放射组学特征,共涉及2 055个指标。采用基于线性SVM的递归特征消除(SVM-RFE)特征选择算法确定最佳特征。使用具有这些最佳特征的5个常见机器学习分类器(QDA、SVCrbf、SVCsigmoid、RFC和Xgboost)开发了一个集成分类器。通过受试者工作特征曲线(ROC)在训练队列和测试队列评估集成分类器区分SCC和AD的性能。[结果] 经过基于统计分析的特征选择,最终获得了来自瘤内和瘤周区域选择的9个最佳特征,包括2个firstorder特征,2个glrcm特征和5个glszm特征。从瘤内和瘤周区域确定的分类模型在预测SCC和AD方面整体性能较好,与5个独立分类器开发的模型相比,集成分类器开发的模型在训练集和测试集中显示了较好的性能。集成分类器在训练集中的AUC为0.87(灵敏度为0.80,特异度为0.84),在测试集中的AUC为0.78(灵敏度为0.72,特异度为0.75)。[结论] 基于CT的放射组学策略从瘤内和瘤周区域提取纹理特征,采用SVM-RFE进行最佳特征选择,并利用集成学习进行分类模型开发,在术前预测区分不同非小细胞肺癌组织学亚型时具有良好的精度和稳定性。 |
英文摘要: |
Abstract:[Objective] To investigate the application value of CT texture analysis in differentiating lung squamous cell carcinoma(SCC) and adenocarcinoma(AD). [Methods] From March 2020 to March 2022, 70 patients with postoperatively diagnosed non-small cell lung cancer (NSCLC) admitted in the First Affiliated Hospital of Xinjiang Medical University were enrolled. Patients were randomly assigned to training set(n=50) and test set(n=20). Seven categories of radiomic features were extracted from the intratumoral and peritumoral regions on CT images of patients, involving a total of 2 055 indicators. The optimal features were determined using a linear SVM-based recursive feature elimination(SVM-RFE) feature selection algorithm. An ensemble classifier was developed using five common machine learning classifiers(QDA, SVCrbf, SVCsigmoid, RFC, and Xgboost) with these optimal features. The performance of the ensemble classifier to discriminate between SCC and AD was evaluated in both the training and testing cohorts by receiver operating characteristic(ROC). [Results] After feature selection based on statistical analysis, 9 best features from intratumoral and peritumoral region selection were finally obtained, including 2 firstorder features, 2 glrcm features and 5 glszm features. The classification models determined from the intratumoral and peritumoral regions performed better overall in predicting SCC and AD, and the model developed with the ensemble classifier achieved better results in both training and test sets compared to the models developed with five independent classifiers performance. The area under ROC curve (AUC) of the ensemble classifier in the training set was 0.87 (sensitivity=0.80 and specificity=0.84), and the AUC in the test set was 0.78 (sensitivity=0.72 and specificity=0.75). [Conclusion] The model based on CT texture deve-loped in the study can preoperatively predict and differentiate between different NSCLC histological subtypes with good precision and stability. |
在线阅读
查看全文 查看/发表评论 下载PDF阅读器 |