欧 笛,姚劲草,李 伟.基于ABUS和机器学习模型对乳腺结节良恶性判断的对比研究[J].肿瘤学杂志,2021,27(12):1001-1005. |
基于ABUS和机器学习模型对乳腺结节良恶性判断的对比研究 |
Differential Diagnosis of Benign and Malignant Breast Nodules Based on ABUS and Machine Learning Model |
中文关键词 |
DOI:10.11735/j.issn.1671-170X.2021.12.B006 |
|
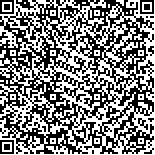 |
中文关键词: 乳腺全容积成像 机器学习模型 乳腺结节 |
英文关键词:ABUS machine learning model breast nodules |
基金项目:国家自然科学基金(82071946);浙江省自然科学基金(LSD19H180001) |
|
摘要点击次数: 667 |
全文下载次数: 104 |
中文摘要: |
摘 要:[目的] 探讨基于乳腺全容积成像ABUS机器学习模型对乳腺结节良恶性的诊断效能。[方法] 收集因“乳腺结节”就诊的164例患者,均行手术治疗且病理诊断明确,采用回顾性研究,根据患者手术病理结果分为良性组和恶性组,对患者进行乳腺ABUS检查后勾画图像,提取影像组学特征,使用四种机器学习方法(LR、FR、SVM和DL)进行独立识别测试,检测DL的阳性预测值、阴性预测值、阳性似然比、阴性似然比等。[结果] 使用LR、RF、SVM和DL对乳腺结节进行良恶性判断的ROC曲线下面积分别为0.592、0.627、0.715和0.737,选取ROC曲线下面积最大的LD模型进行进一步诊断效能分析,当阈值=0.6时,LD识别的约登指数最大(0.44),对应的阳性预测值为69.81%、阴性预测值为77.48%、阳性似然比为3.80,阴性似然比为0.48。[结论] 深度学习在目前使用的机器学习方法中对乳腺结节进行良恶性判断有一定临床诊断意义,未来如果训练集样本数量提高,理论上深度学习方法可以获得更好的识别效果。 |
英文摘要: |
Abstract: [Objective] To explore the application of a full-volume breast imaging(ABUS) machine learning model for the differential diagnosis of benign and malignant breast nodules.[Methods] One hundred and sixty-four patients with breast nodules who underwent surgical treatment were divided into benign group(n=60) and malignant group(n=104) according to the surgical and pathological results. After breast ABUS examination, the image omics characteristics were extracted. Four machine learning methods(LR, RF, SVM and DL) were used for diagnosis of breast nodules, the positive predictive value, negative predictive value, positive likelihood ratio and negative likelihood ratio were evaluated. [Results]The areas under the ROC curve(AUC) of LR, RF, SVM and DL was 0.592, 0.627, 0.715 and 0.737 respectively. The LD model with the largest area under the ROC curve was selected for further diagnostic efficiency analysis. Through calculation, when the threshold=0.6, the Yoden index of LD recognition was the largest(0.44), and the corresponding positive predictive value was 69.81%, the negative predictive value was 77.48%, the positive likelihood ratio was 3.80 and the negative likelihood ratio was 0.48.[Conclusion] Deep learning has certain clinical diagnostic significance in differentiating the benign and malignant of breast nodules in the currently used machine learning methods. In the future, if the number of samples in the training set is increased, the deep learning method may achieve better differentiating effect. |
在线阅读
查看全文 查看/发表评论 下载PDF阅读器 |