|
基于可解释性机器学习方法的甲状腺乳头状癌颈部淋巴结转移预测模型构建 |
Construction of Cervical Lymph Node Metastasis Prediction Model for Papillary Thyroid Carcinoma Based on Interpretable Machine Learning Method |
投稿时间:2025-01-13 修订日期:2025-03-03 |
DOI: |
|
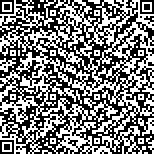 |
中文关键词: 甲状腺乳头状癌 颈部淋巴结转移 LightGBM模型 SHAP值 |
英文关键词:Papillary thyroid carcinoma Cervical lymph node metastasis LightGBM SHAP values |
基金项目:1.广西壮族自治区卫生健康委员会自筹经费科研课题(Z20211602);2.浙江省疾病预防控制科技计划项目 (2025JK137);3.浙江省中医药科技计划项目(2024ZL1261);4.柳州市科技计划项目(2022CAC0229); |
|
摘要点击次数: 10 |
全文下载次数: 0 |
中文摘要: |
[目的]构建和比较多个基于机器学习方法的甲状腺乳头状癌(PTC)颈部淋巴结转移预测模型,筛选出性能最优的模型并提高模型的可解释性。[方法]回顾性分析2021年1月至2023年9月期间,在广西科技大学第二附属医院接受手术治疗的903例PTC患者的临床资料。纳入性别、年龄、肿瘤最大直径、病灶数量及是否存在被膜侵犯等临床病理特征。所有患者随机分为训练集(70%)和验证集(30%)。使用逻辑回归、梯度提升机、随机森林、决策树、神经网络、支持向量机等10种机器学习算法对颈部淋巴结转移的风险进行建模。通过受试者工作特征曲线下面积(AUC)、灵敏度、特异性等指标全面比较各模型性能。同时,通过决策曲线、SHAP值等可视化方法,进一步分析最优模型的效果和可解释性。[结果]在10个机器学习模型中,LightGBM的预测效果最佳。AUC为0.853(95%CI:0.793-0.837),准确率为0.771,F1得分为0.764,敏感度为0.716,特异度为0.799,表现出优异的性能。在决策曲线分析中,LightGBM模型显示出良好的稳定性。[结论] LightGBM模型对甲状腺乳头状癌颈部淋巴结转移构建的预测模型具有良好的预测效果。 |
英文摘要: |
Abstract
Objective
This study aims to construct and compare various machine learning-based predictive models for cervical lymph node metastasis (NLNM) in patients with papillary thyroid carcinoma (PTC), to screen out the models with the best performance and improve their interpretability.
Methods
The clinical data of 903 patients with PTC who received surgical treatment in the Second Affiliated Hospital of Guangxi University of Science and Technology from January 2021 to September 2023 were retrospectively analyzed. The clinicopathological features such as sex, age, maximum tumor diameter, number of lesions and presence or absence of capsular invasion were included. All patients were randomly divided into a training set (70%) and a validation set (30%). Ten machine learning algorithms, including logistic regression, gradient elevator, random forest, decision tree, neural network and support vector machine, were used to model the risk of cervical lymph node metastasis. The performance of each model was compared by the area under the receiver operating characteristic curve (AUC), sensitivity and specificity. At the same time, visualization methods such as decision curve and SHAP value are used to further analyze the effect and interpretability of the optimal model.
Results
Of the 10 machine learning models, LightGBM had the best prediction results. The AUC was 0.853(95%CI:0.793-0.837), the accuracy was 0.771 the F1 score was0.764, the sensitivity was0.716, and the specificity was0.799, showing excellent performance. In the analysis of decision curve, LightGBM model shows good stability.
Conclusion
LightGBM model is effective in predicting cervical lymph node metastasis of papillary thyroid carcinoma.
Keywords: Papillary thyroid carcinoma; Cervical lymph node metastasis; LightGBM; SHAP values |
在线阅读
查看/发表评论 下载PDF阅读器 |
|
|
|