申 帆,王永存,马万瑞,等.基于机器学习预测新诊断喉癌患者的淋巴结转移[J].中国肿瘤,2023,32(12):956-964. |
基于机器学习预测新诊断喉癌患者的淋巴结转移 |
Prediction Models for Lymphatic Metastasis in Newly Dia-gnosed Laryngeal Cancer Patients Based on Machine Learning |
投稿时间:2023-07-18 |
DOI:10.11735/j.issn.1004-0242.2023.12.A010 |
|
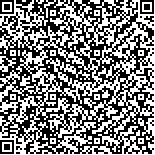 |
中文关键词: 淋巴结转移 机器学习 喉癌 |
英文关键词:lymphatic metastasis machine learning laryngeal cancer |
基金项目:国家自然科学基金(71974040,81273185) |
|
摘要点击次数: 293 |
全文下载次数: 132 |
中文摘要: |
摘 要:[目的] 建立一个机器学习预测模型,可用于预测新诊断喉癌患者的淋巴结转移。[方法]回顾性分析2018—2020年美国国立癌症研究所监测、流行病学和最终结果数据库(SEER)中新诊断喉癌患者的人口统计学变量和临床病理变量,开发基于机器学习的淋巴结转移预测模型。利用ROC曲线下面积大小(AUC)、准确率、召回率、精确率和特异度来评估和比较各机器学习模型的预测性能。[结果] 共纳入4 462例喉癌患者,其中1 444例发生淋巴结转移。年龄、原发部位、临床分级和T分期可以作为独立的风险因素。原发部位、T分期、年龄在淋巴结转移的重要性评估中排前3位。在所有的机器学习模型中,除KNN模型预测效果较差外,Random Forest、Boost、GLM、Neuralnet、Decision Tree这5种模型对于新诊断喉癌患者是否发生淋巴结转移都有较好的预测效果且分类能力近乎相同(在测试集中AUC值均在0.83左右),而准确率、召回率、特异度和精确率则在较小范围内各有优劣。[结论] 本研究构建的机器学习模型可以较好地预测新诊断喉癌患者是否发生淋巴结转移,为临床医生的临床决策提供帮助。机器学习技术有可能帮助喉癌患者淋巴结转移预测模型的开发。 |
英文摘要: |
Abstract: [Purpose] To establish machine learning prediction models for lymphatic metastasis in patients with newly diagnosed laryngeal cancer. [Methods] The demographic and clinicopathological data of newly diagnosed laryngeal cancer patients obtained from the Surveillance, Epidemiology, and End Results database (SEER) during 2018 to 2020 were retrospectively analyzed. On this basis, the prediction models of lymphatic metastasis based on machine learning was developed. Area under ROC curve(AUC), accuracy, recall, specificity and precision were used to evaluate and compare the prediction performance of the established machine learning models. [Results] A total of 4 462 patients with newly diagnosed laryngeal cancer were included in the study, of whom 1 444 occurred lymphatic metastasis. Age, primary site, clinical grade and T stage were independent risk factors for lymphatic metastasis. The primary site, T stage and age were the top three risk factors of lymphatic metastasis. Among all machine learning models except the KNN model, including Random Forest, Boost, GLM, Neuralnet and Decision Tree had good prediction effects (AUC value in the test set was about 0.83), while accuracy, recall, specificity and precision had their own advantages and disadvantages in a small range. [Conclusion] The machine learning models constructed in this study can be used for predicting lymphatic metastasis in patients with newly diagnosed laryngeal cancer, indicating that machine learning technology has a potential to help clinicians for clinical decision making. |
在线阅读
查看全文 查看/发表评论 下载PDF阅读器 |